Justyna Zwolak: Intelligent Quantum Technologies
and Beyond
Justyna Zwolak is a Scientist in the Applied and Computational Mathematics Division at the National Institute of Standards and Technology in Gaithersburg, MD. She received an M.Sc. in Mathematics from The Faculty of Mathematics and Informatics and a Ph.D. in Physics from the Faculty of Physics, Astronomy and Informatics at Nicolaus Copernicus University, in Toruń, Poland. She subsequently was a research associate in the Department of Physics at Oregon State University, at the STEM Transformation Institute at Florida International University, and an assistant research scholar in the Joint Center for Quantum Information and Computer Science at the University of Maryland in College Park, MD.
Her current research focuses on developing machine learning algorithms and artificial intelligence for quantum technologies. In particular, she is investigating methods to automatically characterize and calibrate stable charge configurations in semiconductor quantum dot devices — an appealing quantum computing platform. She is also developing a software suite that enables the modeling of quantum dot devices, training of specialized recognition networks, and — through mathematical optimization — auto-tuning experimental setups. Success in this endeavor will eliminate the need for heuristic calibration and help scale up quantum computing into larger quantum dot arrays. In addition to quantum dot experiments, she is investigating the applicability of machine learning to control, optimize, and stabilize experiments with ultra-cold atoms.
Justyna’s past research pursuits range from quantum information theory to complex network analysis to mathematics and physics education. In particular, she developed novel approaches to characterizing entanglement in quantum systems and delineating the space of quantum states. Using recursive techniques and linear algebra, she proved that classes of linear maps hold certain mathematical properties (positive, but not completed positive, optimal, etc.), which enabled extending so-called entanglement witnesses into high-dimensional composite systems. She also led efforts to employ and develop network and statistical analyses to identify factors that affect student persistence in introductory physics courses. This work resulted in a number of surprising findings (for instance, that social integration is more important than grades in predicting persistence for certain cohorts of students).
Awards and recognition
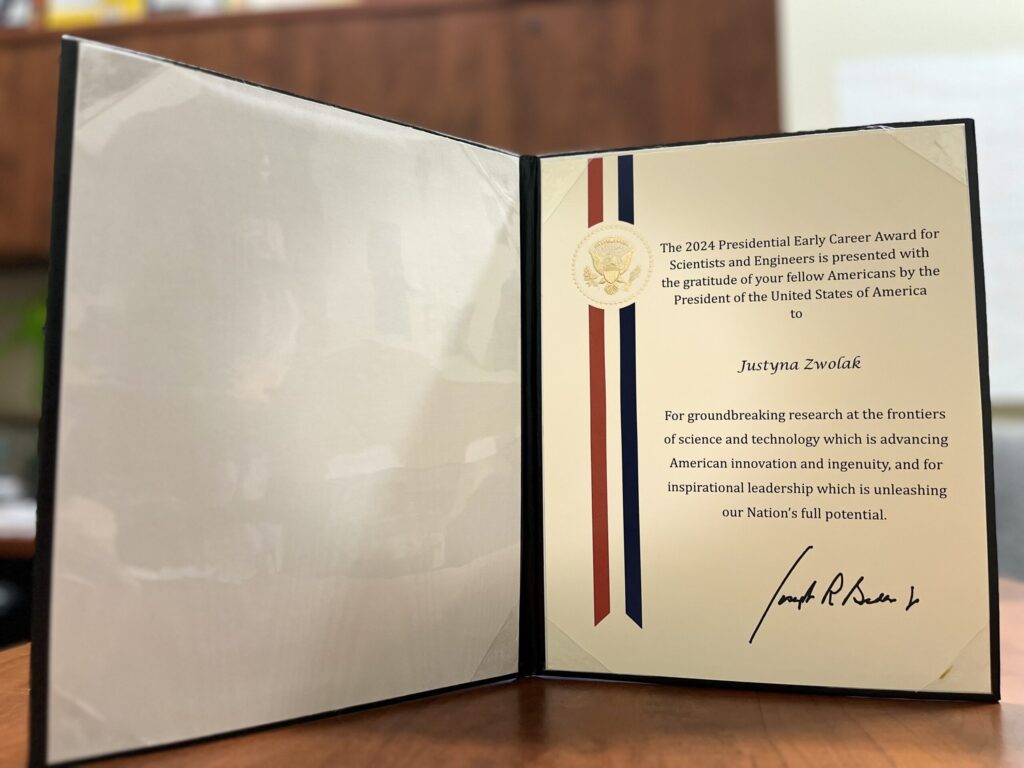
Presidential Early Career Award
for Scientists and Engineers
(January 2025)
“For the development of fundamental and applied advances
at the intersection of machine learning and quantum information science, pushing forward both the state-of-the-art in automating challenging quantum computing experiments and providing
a new foundation for high-dimensional optimization problems”
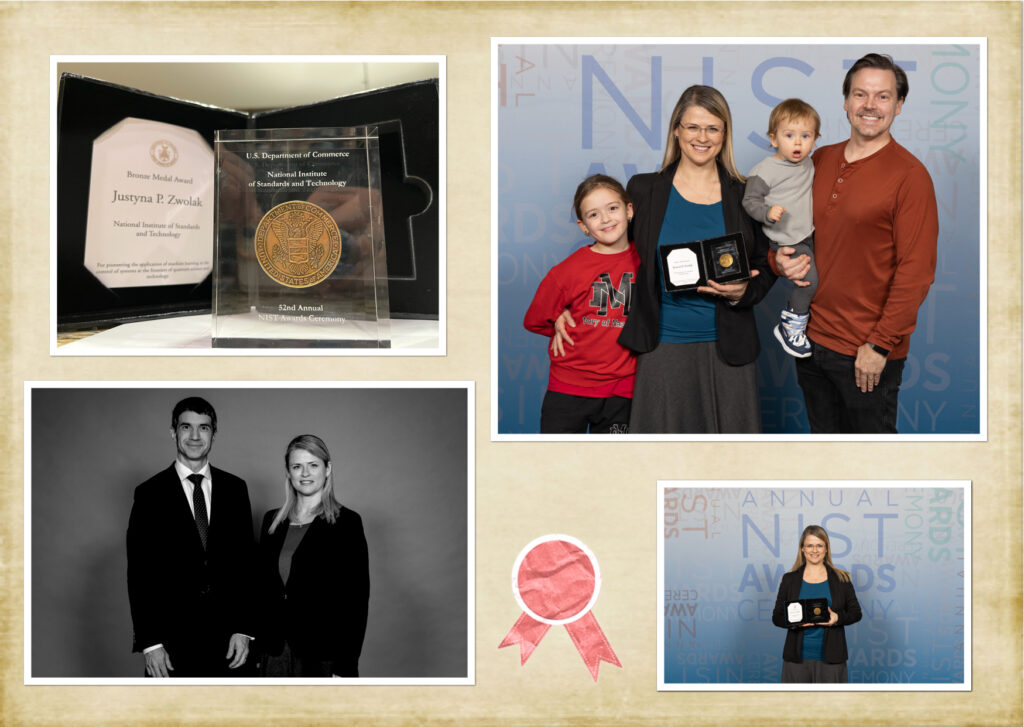
2024 Department of Commerce Bronze Medal Award
(January 2025, Team Award)
“For pioneering the field of machine learning for quantum control.”
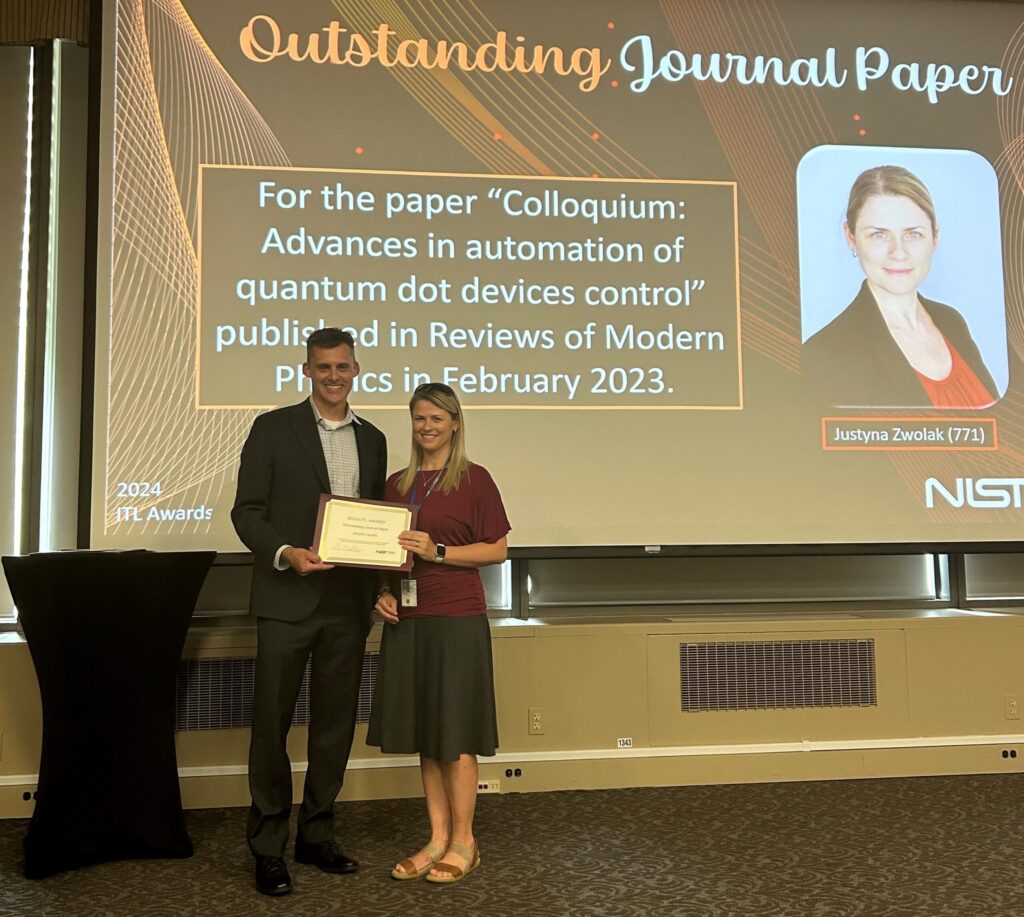
ITL Best Journal Paper of the Year Award
(July 2024)
For your jointly authored paper
“Colloquium: Advances in automation
of quantum dot devices control”
published in Reviews of Modern Physics in February 2023.
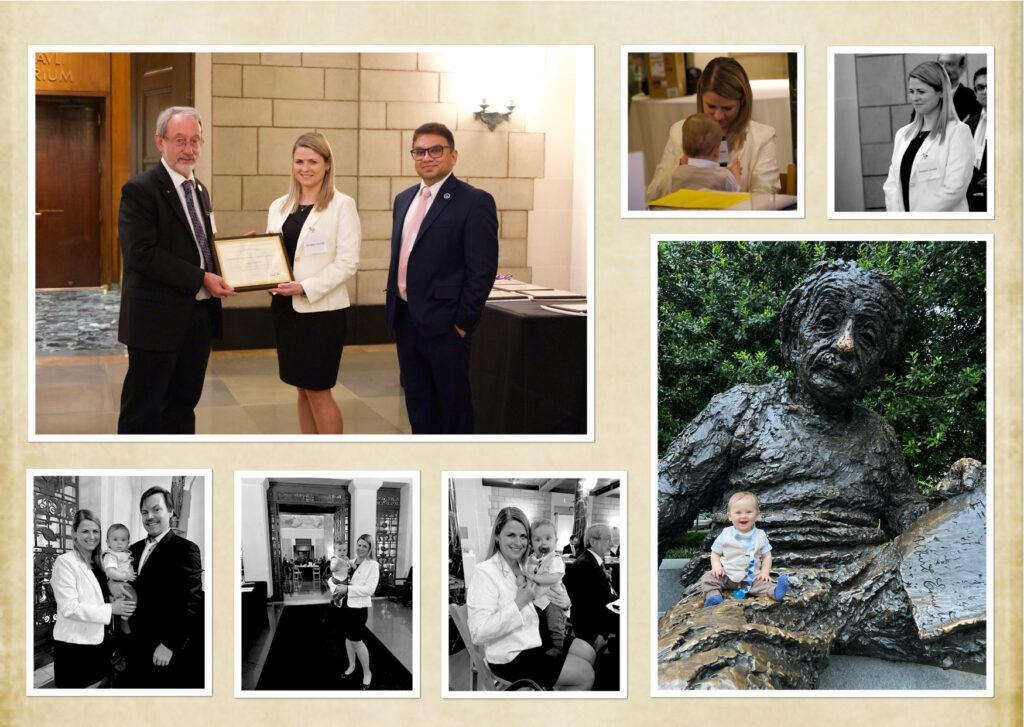
2024 Award for Excellence
in Research in Applied Mathematics
(May 2024)
For outstanding contributions in the application
of machine learning to the control of systems
at the frontiers of quantum science and technology.