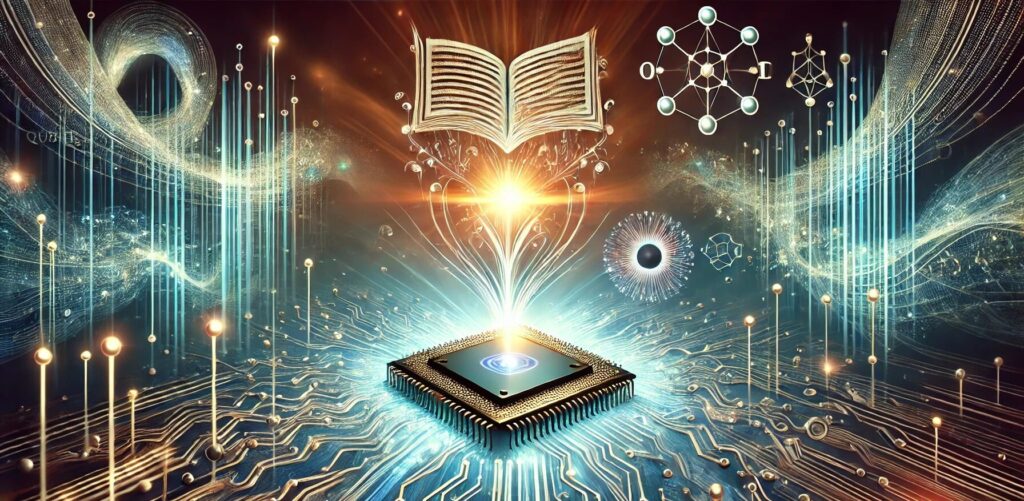
Quantum technology
- A. S. Rao, D. Buterakos, B. van Straaten, V. John, C. X. Yu, S. D. Oosterhout, L. Stehouwer, G. Scappucci, M. Veldhorst, F. Borsoi, and J. P. Zwolak. MAViS: Modular Autonomous Virtualization System for Two-Dimensional Semiconductor Quantum Dot Arrays. arXiv:2411.12516 [to appear in Physical Review X] (2024).
- A. Zubchenko, D. Middlebrooks, T. Rasmussen, L. Lausen, F. Kuemmeth, A. Chatterjee, and J. P. Zwolak. Autonomous bootstrapping of quantum dot devices. Phys. Rev. Appl. 23 (1), 014072 (2025).
- D. Schug, T. J. Kovach, M. A. Wolfe, J. Benson, S. Park, J. P. Dodson, J. Corrigan, M. A. Eriksson, and J. P. Zwolak. Automation of quantum dot measurement analysis via explainable machine learning. Mach. Learn.: Sci. Technol. 6 (1): 015006 (2025)
- J. P. Zwolak, J. M. Taylor, et al. Data needs and challenges for quantum dot devices automation. npj Quantum Inf. 10 (1): 105 (2024).
- D. Schug, T. J. Kovach, M. A. Wolfe, J. Benson, S. Park, J. P. Dodson, J. Corrigan, M. A. Eriksson, and J. P. Zwolak. Explainable Classification Techniques for Quantum Dot Device Measurements. In: Proceedings of the XAI4Sci: Explainable machine learning for sciences workshop (AAAI 2024), Vancouver, Canada [February 26, 2024] (2024).
- D. Schug, S. Yerramreddy, R. Caruana, C. Greenberg, and J. P. Zwolak. Extending Explainable Boosting Machines to Scientific Image Data. In: Proceedings of the Machine Learning and the Physical Sciences Workshop, (NeurIPS 2023), New Orleans, LA, USA [December 15, 2023] (2023).
- J. Ziegler, F. Luthi, M. Ramsey, F. Borjans, G. Zheng, and J. P. Zwolak. Tuning arrays with rays: Physics-informed tuning of quantum dot charge states. Phys. Rev. Appl. 20 (3), 034067 (2023).
- Featured as Editor’s Suggestion in Physical Review Applied.
- J. Ziegler, F. Luthi, M. Ramsey, F. Borjans, G. Zheng, and J. P. Zwolak. Automated extraction of capacitive coupling for quantum dot systems. Phys. Rev. Appl. 19 (5), 054077 (2023).
- Featured as Editor’s Suggestion in Physical Review Applied.
- J. P. Zwolak and J. M. Taylor. Colloquium: Advances in automation of quantum dot devices control. Rev. Mod. Phys. 95(1), 011006 (2023).
- Best Journal Paper of the Year award by NIST/Information Technology Laboratory (2024).
- A. R. Fritsch, S. Guo, S. M. Koh, I. B. Spielman, and J. P. Zwolak. Dark Solitons in Bose-Einstein Condensates: A Dataset for Many-body Physics Research. Mach. Learn.: Sci. Technol. 3 (4): 047001 (2022).
- S. Guo, S. M. Koh, A. R. Fritsch, I. B. Spielman, and J. P. Zwolak. Combining machine learning with physics: A framework for tracking and sorting multiple dark solitons. Phys. Rev. Res. 4(2): 023163 (2022).
- J. Ziegler, T. McJunkin, E. S. Joseph, S. S. Kalantre, B. Harpt, D. E. Savage, M. G. Lagally, M. A. Eriksson, J. M. Taylor, and J. P. Zwolak. Toward Robust Autotuning of Noisy Quantum Dot Devices. Phys. Rev. Appl. 17 (2): 024069 (2022).
- B. J. Weber, S. S. Kalantre, T. McJunkin, J. M. Taylor, and J. P. Zwolak. Theoretical bounds on data requirements for the ray-based classification. SN Comput. Sci. 3 (1): 57 (2022).
- J. P. Zwolak, T. McJunkin, S. S. Kalantre, S. F. Neyens, E. R. MacQuarrie, M. A. Eriksson, and J. M. Taylor. Ray-based framework for state identification in quantum dot devices. PRX Quantum 2 (2): 020335 (2021).
- S. Guo, A. R. Fritsch, C. Greenberg, I. Spielman, and J. P. Zwolak. Machine-learning enhanced dark soliton detection in Bose-Einstein condensates. Mach. Learn.: Sci. Technol. 2 (3): 035020 (2021).
- J. P. Zwolak, S. S. Kalantre, T. McJunkin, B. J. Weber, and J. M. Taylor. Ray-based classification framework for high-dimensional data. In: Proceedings of the Machine Learning and the Physical Sciences Workshop (NeurIPS 2020), Vancouver, Canada [December 11, 2020] (2020).
- J. P. Zwolak, T. McJunkin, S. S. Kalantre, J. P. Dodson, E. R. MacQuarrie, D. E. Savage, M. G. Lagally, S. N. Coppersmith, M. A. Eriksson, and J. M. Taylor. Autotuning of double-dot devices in situ with machine learning. Phys. Rev. Appl. 13 (3): 034075 (2020).
- Featured as Editor’s Suggestion in Physical Review Applied.
- Featured in NIST News: To Tune Up Your Quantum Computer, Better Call an AI Mechanic: New paradigm for “auto-tuning” quantum bits could overcome major engineering hurdle (March 31, 2020).
- S. S. Kalantre, J. P. Zwolak, S. Ragole, X. Wu, N. M. Zimmerman, M. D. Stewart, and J. M. Taylor. Machine Learning techniques for state recognition and auto-tuning in quantum dots. npj Quantum Inf. 5 (1): 6 (2019).
- J. P. Zwolak, S. S. Kalantre, X. Wu, S. Ragole, and J. M. Taylor. QFlow lite dataset: A machine-learning approach to the charge states in quantum dot experiments. PLoS ONE 13 (10): e0205844 (2018).
- J. P. Zwolak and D. Chruściński. Recurrent construction of optimal entanglement witnesses for 2N-qubit systems. Phys. Rev. A 89 (5): 052314 (2014).
- J. P. Zwolak and D. Chruściński. New tools for investigating positive maps in matrix algebras. Rep. Math. Phys. 71 (2): 163–175 (2013).
- S. Michalakis and J. P. Zwolak. Stability of frustration-free systems. Comm. Math. Phys. 322 (2): 277–302 (2013).
- D. Chruściński and J. Pytel*. Optimal entanglement witnesses from generalized reduction and Robertson maps. J. Phys. A: Math. Theor. 44 (16): 165304 (2011).
- D. Chruściński and J. Pytel*. Constructing optimal entanglement witnesses. II. Witnessing entanglement in 4N × 4N systems. Phys. Rev. A 82 (5): 052310 (2010).
- D. Chruściński, J. Pytel*, and G. Sarbicki. Constructing optimal entanglement witnesses. Phys. Rev. A 80 (6): 062314 (2009).
* Papers published under my maiden name. The convention in my PhD group was alphabetical ordering of authors, but I was the primary author of these articles. After I graduated, we no longer adhered to this convention.
Media and Impact
- J. P. Zwolak (March 12, 2025). Marie Skłodowska-Curie: A Legacy of Innovation and Empowerment for Women in Science. Taking Measure: Just a Standard Blog, https://www.nist.gov/blogs/taking-measure/marie-sklodowska-curie-legacy-innovation-and-empowerment-women-science.
- J. P. Zwolak (March 22, 2023). Ada Lovelace: The World’s First Computer Programmer Who Predicted Artificial Intelligence. Taking Measure: Just a Standard Blog, https://www.nist.gov/blogs/taking-measure/ada-lovelace-worlds-first-computer-programmer-who-predicted-artificial.
- Mary Theofanos and J. P. Zwolak (May 16, 2021). Diversity and Inclusivity at NIST. Women in Standards News, https://womeninstandards.org/diversity-and-inclusivity-at-nist/.
- J. P. Zwolak (June 2, 2020). Ebb and Flow: Creating Quantum Dots Automatically With AI. Taking Measure: Just a Standard Blog, https://www.nist.gov/blogs/taking-measure/ebb-and-flow-creating-quantum-dots-automatically-ai.
Social studies
- A. M. Andrews and J. P. Zwolak. NIST Scientific Integrity Program. Annual Report. Natl. Inst. Stand. Technol. Sp. Rep. 1313e2024 (2024).
- R. P. Dalka and J. P. Zwolak. Network analysis of graduate program support structures through experiences of various demographic groups. Phys. Rev. Phys. Educ. Res. 20 (2): 020106 (2024).
- A. M. Andrews and J. P. Zwolak. NIST Scientific Integrity Program. Annual Report. Natl. Inst. Stand. Technol. Sp. Rep. 1313 (2024).
- R. P. Dalka, Diana Sachmpazidi, Charles Henderson, and J. P. Zwolak. Network analysis approach to Likert-style surveys. Phys. Rev. Phys. Educ. Res. 18(2): 020113 (2022).
- E. A. Williams, J. P. Zwolak, R. Dou, and E. Brewe. Linking engagement and performance: The social network analysis perspective. Phys. Rev. Phys. Educ. Res. 15 (2): 020150 (2019).
- R. Dou, and J. P. Zwolak. Practitioner’s guide to social network analysis: Examining physics anxiety. Phys. Rev. Phys. Educ. Res. 15 (2): 020105 (2019).
- An invited article in the Quantitative Methods in PER: A Critical Examination Focused Collection.
- E. M. Smith, J. P. Zwolak, and C. A. Manogue. Isolating approaches: How middle-division physics students coordinate forms and representations in complex algebra. Phys. Rev. Phys. Educ. Res. 15 (1): 010138 (2019).
- C. A. Hass, F. Genz, M. B. Kustusch, P.-P. A. Ouimet, K. Pomian, E. C. Sayre, and J. P. Zwolak. Studying community development: A network analytical approach. In: Proceedings of the Physics Education Research Conference 2018, Washington, DC [August 1-2, 2018], pp. 1–4 (2019).
- R. Dou, E. Brewe, G. Potvin, J. P. Zwolak, and Z. Hazari. Understanding the development of interest and self-efficacy in active-learning undergraduate physics courses. Int. J. Sci. Educ. 40 (13): 1587–1605 (2018).
- J. P. Zwolak, M. Zwolak, and E. Brewe. Educational commitment and social networking: The power of informal networks. Phys. Rev. Phys. Educ. Res. 14 (1): 010131 (2018).
- Featured as Editor’s Suggestion in Physical Review Physics Education Research.
- Featured as a Research Highlight in Nature Physics: “Friendly persistence”, 14: 528 (2018).
- J. P. Zwolak, R. Dou, and E. Brewe. Student perceptions of the value of out-of-class interactions: Attitudes vs. Practice. In: Proceedings of the Physics Education Research Conference 2017, Cincinnati, OH [July 26-27, 2017], pp. 480–483 (2018).
- E. Williams, J. P. Zwolak, and E. Brewe. Physics major engagement and persistence: A phenomenography interview study. In: Proceedings of the Physics Education Research Conference 2017, Cincinnati, OH [July 26-27, 2017], pp. 436–439 (2018).
- K. Pomian, J. P. Zwolak, E. Sayre, S. Franklin, and M. B. Kustusch. Using Social Network Analysis on classroom video data. In: Proceedings of the Physics Education Research Conference 2017, Cincinnati, OH [July 26-27, 2017], pp. 316–319 (2018).
- J. P. Zwolak, R. Dou, E. A. Williams, and E. Brewe. Students’ network integration as a predictor of persistence in introductory physics courses. Phys. Rev. Phys. Educ. Res. 13 (1): 010113 (2017).
- Featured as Editor’s Choice in Science: “The physics of social butterflies”, 356: 282 (2017).
- Featured at phys.org: Social butterflies are more apt to stick with physics (May 15, 2017).
- Featured in FIU News: To keep students interested in physics, have them interact (May 15, 2017).
- R. Dou, E. Brewe, J. P. Zwolak, G. Potvin, E. Williams, and L. H. Kramer. Beyond performance metrics: Examining a drop in students’ physics self-efficacy through a social networks lens. Phys. Rev. Phys. Educ. Res. 12 (2): 020124 (2016).
- J. P. Zwolak and E. Brewe. The impact of network embeddedness on student persistence in introductory Modeling Instruction courses. In: Proceedings of the Physics Education Research Conference 2015, College Park, MD [July 29-30, 2015], pp. 395–398 (2015).
- E. Williams, E. Brewe, J. P. Zwolak, and R. Dou. Understanding centrality: Investigating student outcomes within a classroom social network. In: Proceedings of the Physics Education Research Conference 2015, College Park, MD [July 29-30, 2015], pp. 375–378 (2015).
- E. M. Smith, J. P. Zwolak, and C. A. Manogue. Student difficulties with complex numbers. In: Proceedings of the Physics Education Research Conference 2015, College Park, MD [July 29-30, 2015], pp. 311–314 (2015).
- J. P. Zwolak and C. A. Manogue. Assessing student reasoning in upper-division electricity and magnetism at Oregon State University. Phys. Rev. ST Phys. Educ. Res. 11 (2): 020125 (2015).
- An invited article within the PER in Upper Division Physics Courses Focused Collection.
- J. P. Zwolak and C. A. Manogue. Revealing differences Between Curricula Using the Colorado Upper-Division Electrostatics Diagnostic. In: Proceedings of the Physics Education Research Conference 2014, Minneapolis, MN [July 30-31, 2014], pp. 295–298 (2015).
- J. P. Zwolak, M. B. Kustusch, and C. A. Manogue. Re-thinking the Rubric for Grading the CUE: The Superposition Principle. In: Proceedings of the Physics Education Research Conference 2013, Portland, OR [July 17-18, 2013], pp. 385–388 (2014).